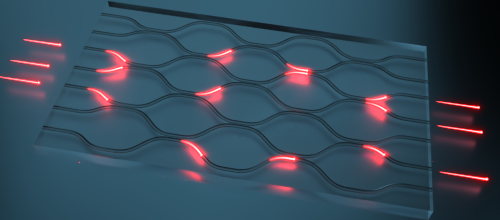
Boson Sampling is a computational problem that requires sampling from the output distribution of a system of n non-interacting bosons after evolution through a linear network. This problem is strongly believed to be hard to solve on a classical computer, being related to the evaluation of matrix permanents with complex entries. Conversely, photons naturally solve the Boson Sampling problem, by directly sampling the outputs of a multi-photon experiment in a linear-optical interferometer. All these ingredients render such problem a promising candidate to obtain an unequivocal quantum computational advantage. This framework motivates the development of technologies that enable precise control of multi-photon interference in large interferometers. We investigate the implementation of Boson Sampling experiments in a photonic platform, by employing femtosecond laser-written circuits that enable three-dimensional structures as well as active reconfiguration capabilities. Scaled up versions of this platform are one of most promising way to demonstrate the computational capability of quantum systems, and may have applications in high-precision measurements, quantum communication or hybrid quantum computation.
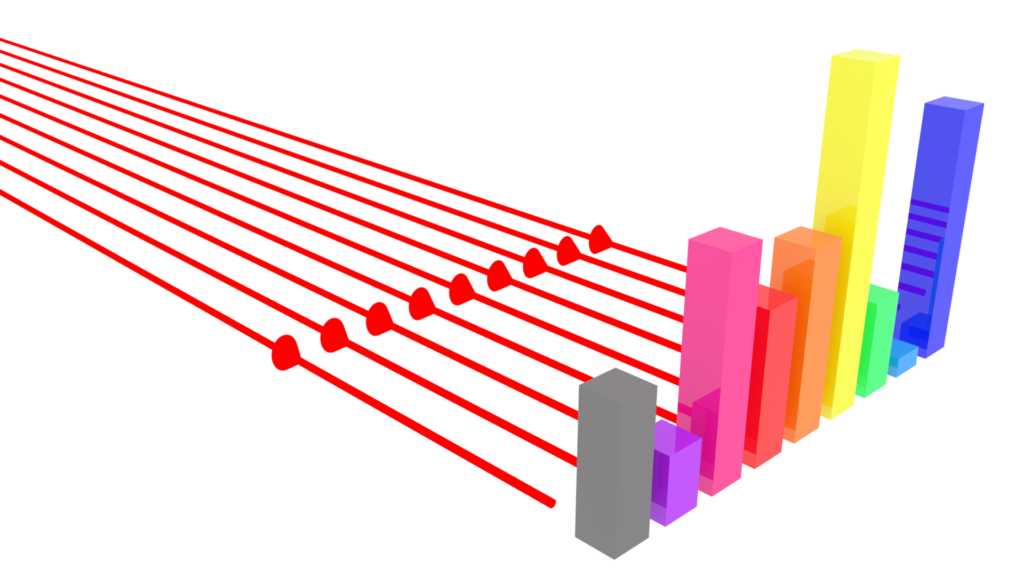
Besides experimental efforts to build large size Boson Sampling apparatus, a fundamental issue is to develop appropriate methodologies to verify the correct function of such class of devices when approaching a classically-hard regime. Indeed, the very complexity of the problem prevents the application of trivial methods involving the calculation of output probabilities (i.e., matrix permanents). Within this context, we are currently investigating a bottom-up approach to assess the correct functioning of a Boson Sampling. In this approach, the idea is to identify classically computable distributions that represent plausible error models for the device operation, and apply suitable methods to exclude that the data were sampled from such distributions. In particular, we are pursuing both a physically-based approach, where such tests are defined from knowledge of the system under investigation, and a computationally-based one, where characteristic patterns are directly recovered from data. In both scenarios, we investigate the adoption of machine-learning techniques as a tool to analyze complex and large datasets.
Selected publications
- D.J. Brod, E. F. Galvao, A. Crespi, R. Osellame, N. Spagnolo, F. Sciarrino. Photonic implementation of Boson Sampling: a review. Adv. Phot. 1, 034001 (2019).
- I. Agresti, N. Viggianiello, F. Flamini, N. Spagnolo, A. Crespi, R. Osellame, N. Wiebe, F. Sciarrino. Pattern recognition techniques for Boson Sampling validation. Phys. Rev. X 9, 011013 (2019).
- T. Giordani, F. Flamini, M. Pompili, N. Viggianiello, N. Spagnolo, A. Crespi, R. Osellame, N. Wiebe, M. Walschaers, A. Buchleitner, F. Sciarrino. Experimental statistical signature of many-body quantum interference. Nature Photonics 12, 173–178 (2018).
- N. Spagnolo, C. Vitelli, M. Bentivegna, D. J. Brod, A. Crespi, F. Flamini, S. Giacomini, G. Milani, R. Ramponi, P. Mataloni, R. Osellame, E. F. Galvao, and F. Sciarrino. Experimental validation of photonic boson sampling. Nature Photonics 8, 615-620 (2014).
- A. Crespi, R. Osellame, R. Ramponi, D. J. Brod, E. F. Galvao, N. Spagnolo, C. Vitelli, E. Maiorino, P. Mataloni, F. Sciarrino. Integrated multimode interferometers with arbitrary designs for photonic boson sampling. Nature Photonics 7, 545 (2013).
Related projects
- QU-BOSS – Quantum advantage via non-linear Boson Sampling
- PHOQUSING – PHOTONICS QUANTUM SAMPLING MACHINE
- PNRR CN1
- QU-DICE – QUantum ranDomness and sImulation proCEssing via non-linear boson sampling
- QUSHIP – Taming complexity with quantum strategies: a hybrid integrated photonics approach